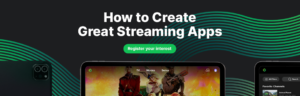
There is only one metric by which you can gauge the success of an IPTV service. It is not subscriber numbers, market share or hours watched: it is revenue. The subscription roll is irrelevant if it isn’t making a profit, so providing the kind of service that engages users, drives organic growth and retains viewers is essential.
The most effective way to do this is through accurate recommendations, but with so many variables to consider, this is increasingly possible only with assistance from machine learning.
The possibilities of machine learning (ML) – and the relevance of its output – is defined entirely by the quality of the data on which it is based.
As Western Digital explains, “The process starts by understanding each viewer: what they are watching now, what they watched before, what they watched next, when they watched, how often they ‘binge’ watch, where they click most on the screen, and more.” Patterns within the data are matched to attributes, like genre, programme length and who features in the content to determine “which videos should be recommended, where they should be placed on the screen, which graphics should be used, and more.”
Machine learning can be paired with artificial intelligence and natural language processing to not only improve the relevance of recommendations made by IPTV services, but the way in which users interact with them. South Korea’s SK Telecom is already running an AI-based IPTV service that allows users to refine their selections by issuing sequential spoken commands, without necessarily specifying content by name. As reported by the Korea Times, results delivered in response to a spoken request for high-definition movies released in the 2000s, for example, can be further refined to include only free options.
Last November, SK Telecom announced plans to overhaul the service, and add AI-based curation features, which would “come up with customised content after analysing subscribers’ TV viewing history”, reports the Korea Herald.
The two data types required by the machine learning algorithm are fundamentally different. One – the metrics about the content on offer – is static, but the other – user behaviour – is live and constantly changing. The IPTV providers that derive the greatest benefit from the possibilities of machine learning, and its ability to deliver a loyal subscriber base, will be those that develop the most comprehensive system for gathering user metrics and scrubbing their database of stale data that no longer applies to its changing demographic.
The most valuable data is temporal, or time based. Freya Rajeshwar, who worked at the Society of Motion Picture and Television Engineers before moving to Google Play, Movies & TV, explains that “IPTV is very Temporal Analytics driven. Some additional analytics are based on Metadata which is primarily text, but the majority of it is behavioural data which is temporal… a typical season of a network TV show is almost exactly a million frames. Each of those 1M+ frames is a reference point for data about user behaviour. And while website analytics may have numerous elements, it is rare that a user has the option to stop, pause, rewind, fast forward, increase volume, decrease volume, mute, etc, times a million frames that a TV series will have. IPTV collective user data nearly always exceeds the size of the video files with the depth and volume of the data that is recorded.”
Building a machine learning system to underpin an IPTV service therefore requires significant investment in the back end, which will collate, parse and analyse the temporal data. Such an investment might not be obvious at the outset, but it could make the difference between a service that can grow its audience and has the data it needs to offer real value by developing additional services, and one that can compete on little other than price.
Machine learning also requires a degree of feedback. How does the back end know whether the recommendations it is making, on the basis of the temporal data it is analysing, are successful? The most obvious metric is whether the viewer performed the action that the IPTV provider wanted.
“Explicitly determining the outcome enables the ML algorithm to make a better guess at the next iteration of the function, thus developing more accurate predictions as time progresses and more data becomes available. This is the concept of learning,” noted Tony Orme at The Broadcast Bridge. “Some parts of television are better suited to ML than others. For example, using ML to discover the viewing habits of OTT delivery to mobile phones is ideally suited to ML as there is a definite outcome; the viewer either watches the program or they do not. Feedback from the mobile device’s viewing software will provide this.”
On a broader scale, Google is using machine learning to make decisions that would previously have been in the hands of a human engineer. The company’s Adam Handman explains how it is, “leveraging machine learning to make better transcoding decisions, to automate live to VOD, to automate clipping, to enhance metadata, to be able to run the content through a speech-to-text engine, or a transcription service, to generate a text transcript that can enhance the metadata, to make better monetisation decisions. Leveraging machine learning to be able to serve up a more targeted ad.”
The possibilities are, seemingly, endless – and as computers get more powerful, and storage prices fall yet further, the temptation will be to gather more data, whether fixed or temporal, in a drive to deliver more effective behavioural nudges. This will only be effective if IPTV providers already know what a successful outcome would constitute. The key, then, may not be to gather every data point possible but to ask, first, what result they hope to deliver, and then to consider which data they require to service that goal. Everything else is just noise.
Thank you for engaging with our LinkedIn post.
We would love to share further market insights, technical whitepapers and product information.
Need to ask us a question or get in touch with our sales team?
Please use the form below to contact us
and one of our team will be delighted to get back to you within a working day.
Alternatively, you can email grow@smartlabs.tv
Please contact me to schedule a demonstration of SmartCARE: